Federated learning
Investigating accuracy in federated learning
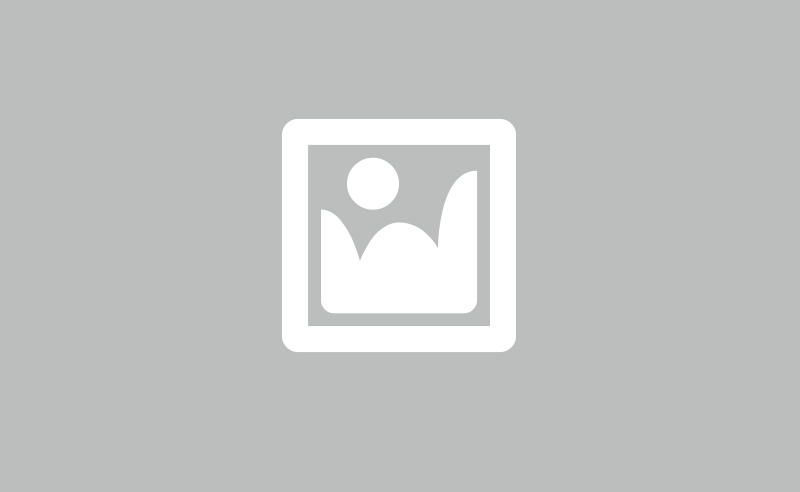
Dr Muhammad Rizwan
Project description
For data privacy reasons, many organisations are adopting 'federated learning' technologies, where machine learning models are trained based on data held by clients on remote devices. Many different approaches have been suggested, with different tradeoffs between computation, communication, accuracy and privacy. Increasing amounts of effort are put into achieving seemingly small improvements in accuracy of these models.
This project is to survey the use of federated learning in practice, as reported in publications and reports, and to provide an overview of the state-of-the-art. In particular, it will consider the use cases in which small changes in computation, communication, accuracy or privacy have a significant impact on the efficacy of method in addressing the use case. The output will also be to provide recommendations and identify opportunities for more research.
Working with our leading cybersecurity team, the findings from this internship will help to underpin teaching practice within the WMG cybersecurity programmes, helping to support the fast-paced nature of research-led teaching practice in cybersecurity.
Duties and responsibilities
- Perform research into published work
- Summarise findings in a report
- Present the findings to stakeholders
Required skills
- Good knowledge of machine learning concepts and techniques
- Good knowledge of Python
- Clear written and verbal communication skills
Acquired skills
- Experience in performing a literature review
- Experience in distilling information into key findings
- Experimentation and communication of results to stakeholders
- Engagement with stakeholder
Apply for this project
Use the form below to apply for this project.
You must be signed in to submit your application and to receive a confirmation email.
This form is closed and is no longer accepting any submissions. Thank you for your time.