Data Science and Machine Learning: The Fundamentals | Short course at the Summer School at Warwick University
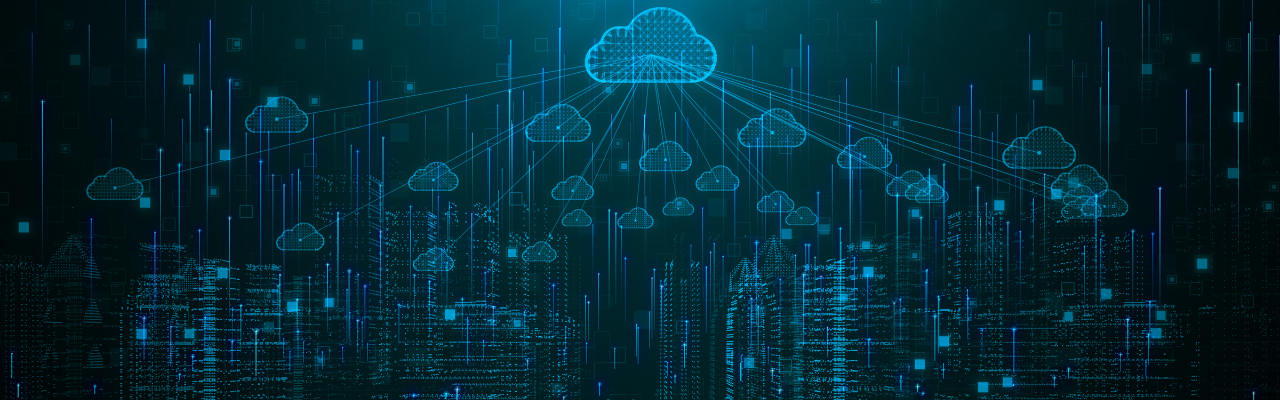
Data Science and Machine Learning: The Fundamentals
The most important aspect of computer science is problem solving, an essential skill for life.
Data Science is concerned with how to gain knowledge from the vast volumes of data generated daily in modern life, from social networks to scientific research and finance, and proposes sophisticated computing techniques for processing this deluge of information. In parallel, Machine Learning is concerned with the development of analytical models and algorithms to learn from data and make accurate predictions.
This course addresses fundamental aspects of Data Science and Machine Learning, e.g., analytical models to represent and understand the data, efficient algorithms to manipulate and extract relevant knowledge, and corresponding models to understand their overall performance and limitations.
In particular, students study the design, development and analysis of software and hardware used to solve problems in a variety of business, scientific and social contexts. During this course, students will study techniques for how to go from raw data to a deeper understanding of the patterns and structures within the data, to support making predictions and decision making. Students would be expected to have some basic knowledge of linear algebra and calculus.
Key Information
Level: Introductory to intermediate
Fees: Please see Fees page
Teaching: 60 hours
Expected independent study: 90 hours
Optional assessment: Dependent on course
Typical credit: 3-4 credits (US) 7.5 ECTS points (EU) - please check with your home institution
This course can also be combined with our Exploring British Culture week - find out more.
Data Analytics involves being about to go from raw data to a deeper understanding of the patterns and structures within the data, to support making predictions and decision making. The course will cover a number of topics, including:
- Introduction to Data Science and Machine Learning: motivating successful analytic examples (Walmart, Google, and Twitter), introducing Supervised, Unsupervised, and Reinforcement Learning, measuring performance / regret, fundamental limits (the No-Free-Lunch-Theorem and Bias);
- Probability recap, e.g., sample spaces, random variables, distributions, heavy-tails, quantiles, Q-Q plots, Bayes, correlation;
- Statistics recap, e.g., hypothesis testing, chi-square distributions, density estimation (MoM and MLEs), confidence intervals and application to voting;
- Stochastic bandits as a fundamental example of Reinforcement Learning: naïve Explore-then-Exploit strategy and UCB bounds;
- Regression: linear regression, least squares, logistic regression - Predicting new data values via regression models. Simple linear regression over low dimensional data, regression for higher dimensional data via least squares optimization, logistic regression for categoric data;
- Matrices: Linear Algebra, SVD, PCA - Matrices to represent relations between data, and necessary linear algebraic operations on matrices. Approximately representing matrices by decompositions (Singular Value Decomposition and Principal Components Analysis). Application to the Netflix prize;
- Classification: Trees, NB, Support Vector Machines, Kernel Trick - Building models to classify new data instances. Decision tree approaches and Naive Bayes classifiers. The Support Vector Machines model and use of Kernels to produce separable data and non-linear classification boundaries. The Weka toolkit;
- Clustering: hierarchical, k-means, k-center - Finding clusters in data via different approaches. Choosing distance metrics. Different clustering approaches: hierarchical agglomerative clustering, k-means (Lloyd's algorithm), k-center approximations. Relative merits of each method;
- Basic tools: command line tools, plotting tools, programming tools - The wide variety of tools available to work with data, including unix/linux command line tools for data manipulation (sorting, counting, reformatting, aggregating, joining); tools such as gnuplot for displaying and visualizing data;
- A number of hands-on exercises involving real data and to be solved in either the Weka toolkit, Python, or R.
Please note changes to the syllabus and teaching team may be made over the coming months before exact set of topics are finalised.