Complete thermodynamic description of alloys to extreme pressure and temperature
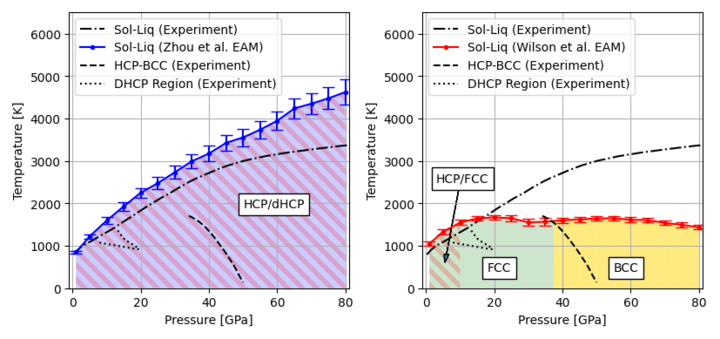
Phase diagrams calculated using nested sampling with two existing magnesium models, compared to experiments,
highlighting discrepancies even at moderate conditions. In this project, highly accurate data-driven models will
be generated to describe metals at extreme conditions which are inaccessible to conventional experimental techniques.
Student: Vincent FletcherLink opens in a new window
Supervisors: Albert Bartók-PártayLink opens in a new window and Livia Bartók-PártayLink opens in a new window
Summary
The aim of my project is to develop a first-principles theoretical and computational scheme that provides a complete thermodynamic description of alloys from ambient to extreme conditions. By 'complete' I mean a technique that is able to compute the Helmholtz free energy of the system, which can then be used to determine other properties such as the equation of state, and therefore phase boundaries, together with properties across boundaries such as latent heats.
Thermodynamic properties, especially in the high-temperature regime require extensive sampling, making application of ab initio calculations - which are highly desirable due to their accuracy and transferability - computationally unfeasible. Surrogate models based on machine learning approaches have a high potential in these applications, but are challenging to fit due to the complex energy landscape of multi-component alloy systems. Another challenge lies in the identification of the thermodynamically stable phases at high temperatures and pressures in regions of the phase diagram that have not been explored experimentally.
This project will bring together phase discovery methods, thermodynamics calculations, machine learning to fit interatomic potentials, and the generation of ab initio databases. Random structure search (RSS) will be used, combined with thermodynamic integration, using Atomic Cluster Expansion (ACE) and Gaussian Approximation Potentials (GAP) fitted from Density Functional Theory (DFT) data. The use of the nested sampling (NS) method will also be explored, as a stand-alone method to determine the phase diagram without prior knowledge of the stable phases. The database creation will be augmented by nested sampling, that will automatically generate the relevant liquid and high-pressure solids.
Key Words:
Machine Learning, Database Generation, Generative Learning, Gaussian Approximation Potentials (GAP), Atomic Cluster Expansion (ACE), Density Functional Theory (DFT), Ab-Initio, Nested Sampling, Phase Diagrams, Crystal Structures, High Performance Computing (HPC), Thermodynamics, Statistical Mechanics, Chemistry, Physics, Programming