Charting a course towards new light-activated molecules
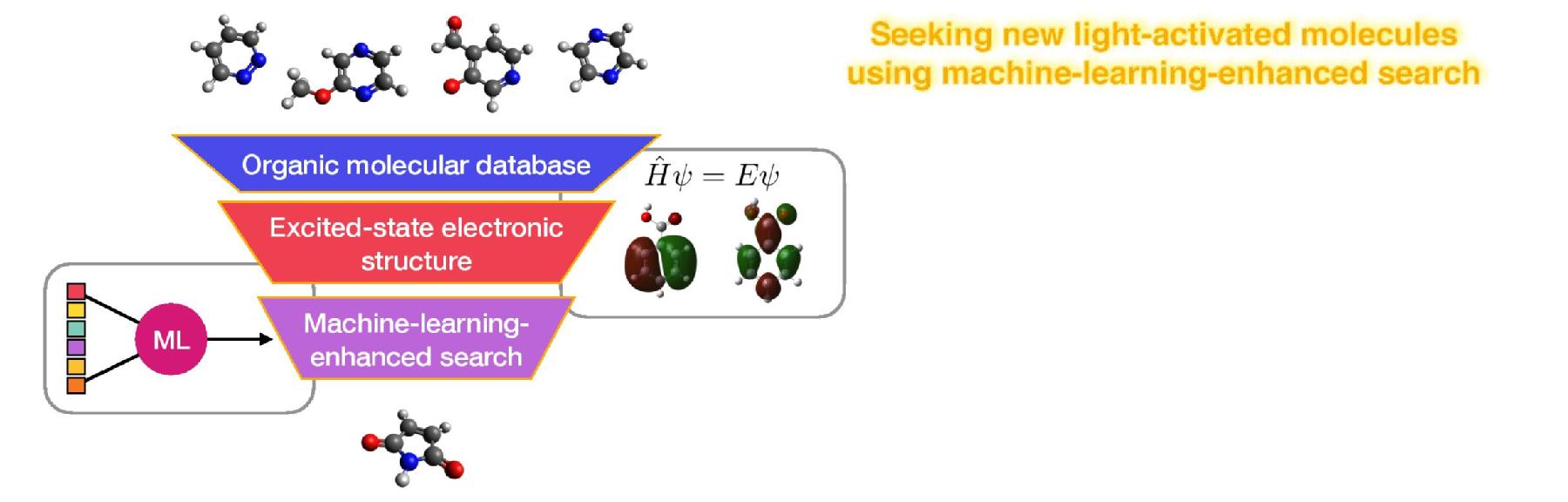
Supervisors: Scott Habershon, Nicholas Hine and Albert Bartok-Partay
Summary:
Many small molecules absorb light. Predicting what happens to them next is a challenging task for computer simulations, but if we could solve this problem we would have a new route to designing new fluorophores for medical diagnostic imaging, new photocatalysts for green synthesis, or new chromophores for harvesting the sun’s energy in solar cells. In this project, we aim to address this challenge by combining excited-state electronic structure calculations and machine-learning to build new predictive models to help us search the entire space of small organic molecules to identify useful (and previously-unknown) light-activated molecules.
Background:
Photofunctional molecules are critical components of important biological and technological systems, including photocatalysts for green organic synthesis, fluorophores for bio-imaging applications, and chromophores for light-harvesting in organic solar cells. However, the emergence of photofunctional behaviour depends sensitively on both the structural and electronic properties of a molecule - features such as the manifold of excited-state energies, inter-state couplings, excited-state minima and conical intersections all play a role in determining what happens to a molecule after it absorbs light.
Correlating these important photophysical features with molecular structure remains an enormous challenge standing in the way of direct or inverse design of new photoactive molecular species for applications in photocatalysis and sensing. Small molecular structural changes can lead to large and abrupt changes in photochemical response - suggesting that better insight into photochemical structure/function relationships are required.
In this project, we will use a combination of excited-state electronic structure, machine-learning, and solvent-environment modelling to address this challenge. Using excited-state electronic structure calculations for molecules drawn from large databases, we will develop machine-learning models to accurately predict photochemical behaviour of small organic molecules; we will then deploy generative models and/or systematic database searches to identify new molecules with targeted photo functionality, notably high fluorescent quantum yield (e.g. acting as imaging fluorophores) or ultrafast ground-state decay (e.g. acting as molecular sunscreens).
Links to HetSys Training
While enormous databases of organic molecules are available, this project requires drawing together different simulations approaches into a new, coherent, integrated workflow merging databases, excited-state electronic structure, machine-learning and solvent-effect prediction. This is a long-term challenge requiring significant software engineering - and the HetSys training is essential to enable the student to integrate the disparate simulation components.
This project combines methods from excited-state chemistry and physics with machine-learning analysis. There is also potential to expand collaborators to organic chemists to synthesise new organic photoactive molecules.
Predicting how molecular structure influences photochemistry is a huge challenge, requiring development of: (i) better ways of capturing structural trends in photochemistry using molecular/electronic descriptors, (ii) better account of uncertainties in predicted photo-behaviour. These challenges are exacerbated by inaccuracies in excited-state electronic structure, which must also be accounted for in accurate predictions of photochemical behaviour.
In summary - integration of databases, machine-learning models, generative models, and excited-state electronic structure calculations will require a “joined up” approach to software development to generate a useful computational pipeline to deliver objectives.