Machine learning multiscale simulation of photoconductivity in correlated oxides
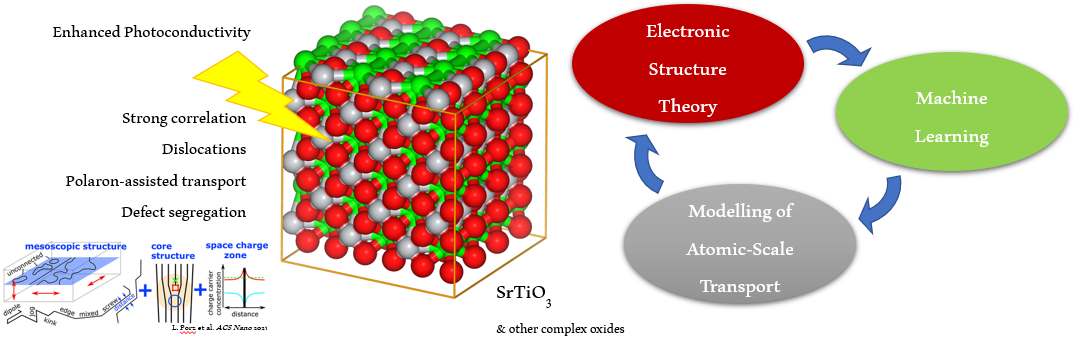
Supervisors: Nicholas Hine, Reinhard Maurer and Marin Alexe
Summary:
Predicting, explaining and modelling novel behaviours of quantum materials requires a combination of theoretical insight with state-of-the-art multiscale modelling. In the case of complex oxides, displaying both strong electronic correlation and a diverse range of extended and point defects, traditional electronic structure methods encounter severe challenges when trying to model key properties such as photoconductivity and bulk photovoltaic effects. Fortunately, the extraordinary speed and power of machine-learned interatomic potentials provides a brand-new way to gain insight into these systems. This project will design and build multiscale models to understand photoconductivity in SrTiO3, particularly enhancement associated with dislocation cores.
Background:
The transport properties of traditional semiconductors can be understood through well-established theoretical methods. Dislocations, grain boundaries and point defects typically reduce conductivity in such materials. The situation can be very different for complex oxide materials such as SrTiO3. In these systems, defects are often what leads to desired functionality in the first place. Recently, highly unexpected behaviour of photoconductivity has been observed at dislocations in SrTiO3 [1]: different slip systems display different photoconductivity, by several orders of magnitude, and a photovoltaic effect enabled by dislocation-surrounding strain fields was observed, demonstrating great scope for tailored, anisotropic photoelectric functionality.
The main barrier to understanding is that complex oxides exhibit strong electron correlation, localized electrons, and the formation of polarons, which provide a challenge for existing electronic structure theory and conventional transport theories. In such materials charge carriers are strongly correlated with lattice vibrations, and thus cannot be described with traditional band theory [2]. Additionally, the intrinsic scale of dislocation defects, spanning hundreds to thousands of atoms, precludes the outright application of expensive correlated electronic structure methods.
This project addresses this challenge by establishing a multiscale simulation framework to study conductivity in nanostructured correlated oxides. Machine learning methods will provide capabilities to sample the structure and lattice dynamics of defects at realistic scales, whereas Hilbert space projection techniques [3] will provide paths to map correlated electronic structure onto viable models to simulate polaron formation and transport.
Links to HetSys Training
This project will involve 1) large-scale electronic structure calculations, elucidating behaviour at dislocations and providing training data for 2) physics-informed machine-learned interatomic potentials (MLIPs) enabling fast and robust predictions of structure, dynamics, and electronic structure, enabling development of 3) of effective model Hamiltonians based on ‘downfolding’ onto few electronic states to describe polaron transport and photoconductivity.
The student will need to integrate the three modelling strands, each at different length and timescales, with experimental data from the Functional Electronic Materials group. An inherently interdisciplinary challenge will be investigated, which if overcome has the potential to generate considerable new understanding in a regime in which ab initio modelling has not been particularly successful to date.
Experimental data will constrain modelling to some extent, but the key to predictive power will be to understand how errors propagate from electronic structure (eg dependence of bandgap & electronic polarisability on DFT methods) to MLIPs & single-particle hamiltonians (choices of basis functions can limit accuracy) and finally to effective low-energy models.
The three-level multiscale model being proposed for polaron transport will require a robust framework for reliable workflows. The Hine and Maurer groups have extensive experience developing and documenting such workflows.
References:
[1] Kissel et al Adv. Mater. 34 2 (2022)
[2] Zhou and Bernardi, Phys Rev Research 1 033138 (2019)
[3] Westerhout et al 2D Mater 9 014004 (2022).