Research internship projects
The projects below are illustrative of the type of project you might work on for either the URSS scheme or the Warwick Statistics Internship. This list is a small subset of the projects on offer. If you are interested in a project below, please contact the relevant supervisor. For other projects, please browse our list of our academic staffLink opens in a new window and take a look through their research interests. Once you have identified potential supervisors, please contact them to discuss projects. URSS applicants must apply with a specific project and supervisor. This is not essential for applicants to the Warwick Statistics Internship, but it is advantageous to have discussed projects (informally) with a prospective supervisor before you apply.
Dr Richard Everitt 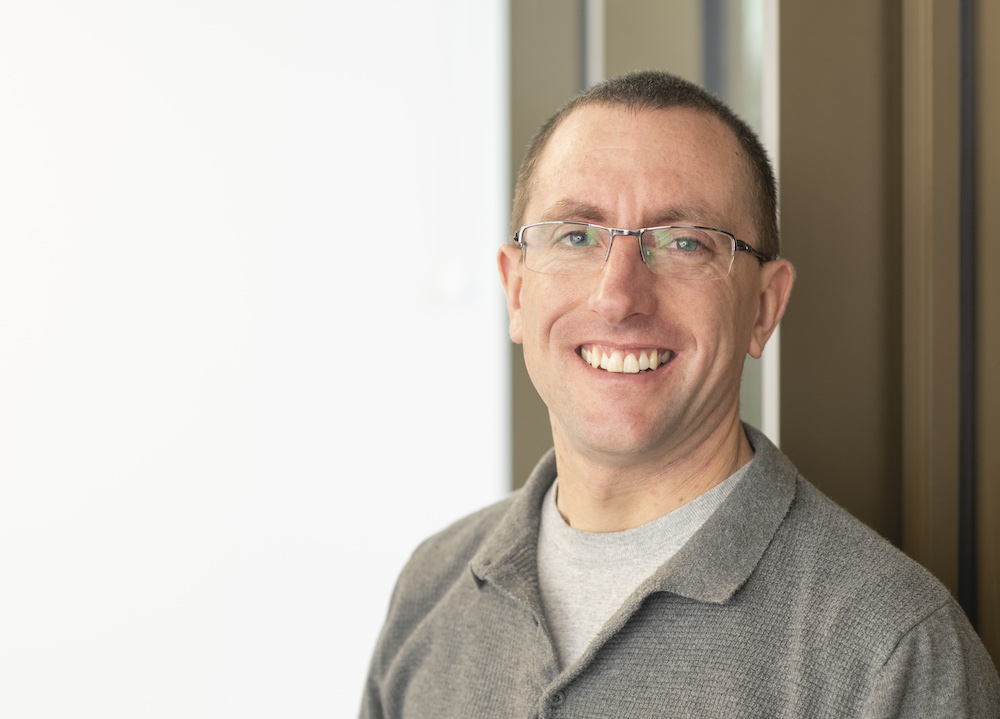
Approximate Bayesian computation for individual based models
Estimating bowler ability in cricket
Active subspaces using sequential Monte Carlo
Prof. Ioannis Kosmidis 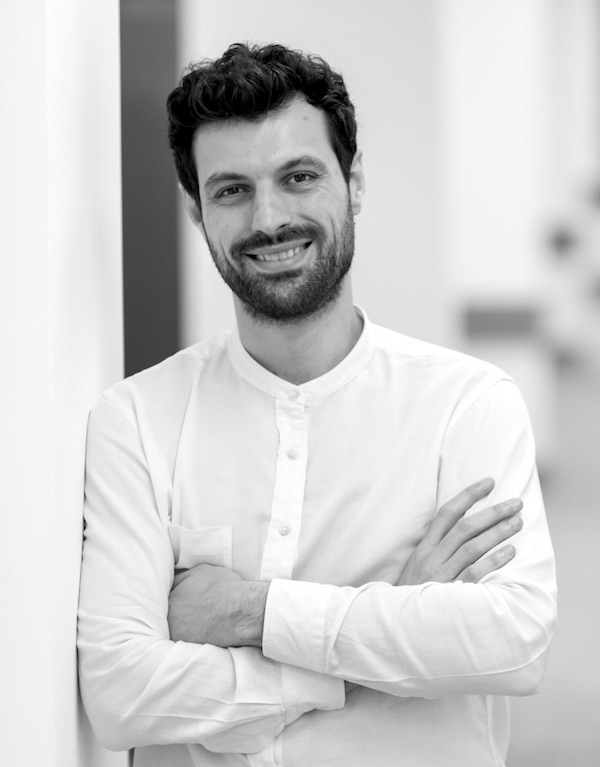
High-dimensional logistic regression
Item-response theory models and politics: How liberal are the members of the US House?
Dr Martyn Parker
Learning analytics and natural language processing
Learning analytics aims to use data about learning and its contexts to optimise education and the spaces in which it occurs. As a field, it intersects data science, statistics, human-centred design, and educational research. Typical methodologies include:
- Descriptive analytics. Use of data aggregation and mining to evaluate trends and metrics over time.
- Diagnostic analysis. Insights into why outcomes occurred.
- Prescriptive analysis. Provide recommendations on outcomes.
Data sources can include quantitative and qualitative data sources.
The challenges are complex and current tools often lack theoretical rigour and focus on data presentation rather than applying formal data analysis principles to support evidence-based decision-making, for example, diagnostic analysis needs to be stronger. Furthermore, qualitative data analysis should take advantage of current advances in natural language processing.
This project aims to offer the opportunity to
- critically review of current learning analytics tools and methodologies.
- Identify gaps in current diagnostic analysis approaches and tools.
- Apply rigorous statistical and data analysis techniques to create tools that provide rigorous diagnostic insight and justifiable prescriptive analytics.
- Apply natural language processing techniques to create new descriptive and diagnostic analytics.
Required skills:
- Ability to analyse a range of both quantitative and qualitative data sets using a range of statistical tools.
- Knowledge of, or desire to learning natural language processing techniques and apply them to a developing area.
- Strong interest is application of statistical and data analysis to learning.
- Strong statistical programming skills that also focus on human-centred interactions, for example, useability
A key output will be a theoretically justified learning analytics tool. Since learning analytics data sources contain sensitive information, this project uses illustrative data sets. These data sets illustrate typical inputs into the learning analytics process but are not derived from and do not contain “live” data. Consequently, there are no GDPR or ethical considerations.
References:
K G, S., Kurni, M. (2021). Introduction to Learning Analytics. In: A Beginner’s Guide to Learning Analytics. Advances in Analytics for Learning and Teaching. Springer, Cham. https://doi.org/10.1007/978-3-030-70258-8_1
Guzmán-Valenzuela, C., Gómez-González, C., Rojas-Murphy Tagle, A. et al. Learning analytics in higher education: a preponderance of analytics but very little learning?. Int J Educ Technol High Educ 18, 23 (2021). https://doi.org/10.1186/s41239-021-00258-x
Clow, D. (2012). The learning analytics cycle: closing the loop effectively.
Siemens, G., & d Baker, R. S. (2012, April). Learning analytics and educational data mining: towards communication and collaboration. In Proceedings of the 2nd international conference on learning analytics and knowledge (pp. 252-254). ACM.
Shinji Watanabe, Jen-Tzung Chien. (2015) Bayesian speech and language processing Cambridge University Press.
Dan Jurafsky, (2009) Speech and language processing: an introduction to natural language processing, computational linguistics, and speech recognition, Pearson Prentice Hall
Thomas K. Landauer, Danielle S. McNamara, Simon Dennis, Walter Kintsch (Eds), (2007) Handbook of Latent Semantic Analysis, Taylor Francis: New York. https://doi.org/10.4324/9780203936399
CRAN (2021), CRAN Task View: Natural Language Processing, Maintainer: Fridolin Wild, Version 20th October 2021. Available online: https://cran.r-project.org/view=NaturalLanguageProcessing
Rahil Shaiky, (2018) Gentle Start to Natural Language Processing using Python, Published in Towards Data Science, available online: https://towardsdatascience.com/gentle-start-to-natural-language-processing-using-python-6e46c07addf3
Natural language processing: theory and practice
Natural language processing (NLP) combines elements of statistical theory, artificial intelligence (machine learning/deep learning) to understand document contents including language nuances. This project investigates the both the theoretical background and practice implementation for text-based analysis. The theory will cover data pre-processing and different statistical models that can be used to develop language insights. Initial statistical approaches will examine how to provide statistical insights into language data. Time permitting, there is the opportunity to look at probabilistic models used to predict the next word in a sequence, given the preceding words. Practical implementation may include developing tools that apply the theory to data (for example, web pages or downloaded data sources) to organise, analyse, and categorise the information contained in these documents. In the wider context, businesses might apply NLP to customer reviews or feedback to help understand contextual elements, provide insights, detect fake reviews and help develop solutions to key customer-facing issues.
Key outcomes may include:
- Review, synethesis, evaluate and report the investigation of the theory of natural language processing. This would include areas such as tokenisation, lemmatisation, parsing, segmentation, stemming, entity recognition, classification, and semantic analysis.
- Develop a tool that accept as input a range of natural language data sources, process these inputs by applying the theory from part 1, and provides flexible ways to interpret the inputs.
The tool may be able to accept quantitative data and quantitative data that contextualises the quantitative data.
Required background. You must be able to develop tools and visualisations using for example Python/R. You will need to know or be able to learn relevant statistical theory suitable for the statistical modelling techniques employed.
References
Rahil Shaiky, (2018) Gentle Start to Natural Language Processing using Python, Published in Towards Data Science, available online: https://towardsdatascience.com/gentle-start-to-natural-language-processing-using-python-6e46c07addf3
CRAN. (2021) CRAN Task View: Natural Language Processing, Maintainer: Fridolin Wild, Version 20th October 2021. Available online: https://cran.r-project.org/view=NaturalLanguageProcessing
Luiz Felipe de Almeida Brino. (2019) Natural Language Processing with R. Version 3rd June 2019. Available online: https://rpubs.com/LuizFelipeBrito/NLP_Text_Mining_001
Francois Chollet. (2022) Deep Learning with Python (2nd ed.). Manning
Kenneth Church. (2014) Statistical Models for Natural Language Processing, in Ruslan Mitkov (ed.), The Oxford Handbook of Computational Linguistics, 2nd edn, Oxford Handbooks (2022; online edn, Oxford Academic, 1 Apr. 2014). Available online https://doi.org/10.1093/oxfordhb/9780199573691.013.54.
Shinji Watanabe and Jen-Tzung Chien. (2015) Bayesian speech and language processing Cambridge University Press.
Dan Jurafsky. (2009) Speech and language processing: an introduction to natural language processing, computational linguistics, and speech recognition, Pearson Prentice Hall
Dr Simon Spencer 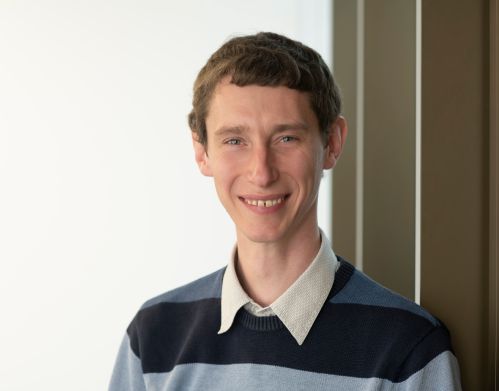
Simulations for the whole family (of quasi-stationary distributions)
If a Markov process has an absorbing state (reached with probability one in finite time) then the stationary distribution is boring – all the mass falls on the absorbing state. However, if we condition on the process not having reached the absorbing state yet then a so-called quasi-stationary distribution may exist. In fact, there can be infinitely many such quasi-stationary distributions for the same process. The birth-death process is a relatively simple model that has an infinite family of quasi-stationary distributions. One is straightforward: a so-called “low energy” distribution with finite mean, and all others are more exotic, “high energy” distributions with infinite mean. In this project we will look to find ways of simulating from the quasi-stationary distributions of the birth death process, and from the “high energy” distributions in particular. Then, we will look to apply these simulation techniques to more complex models in which the family of quasi-stationary distributions is currently unknown. This project will involve programming in the statistical programming language R.
Key reference: Adam Griffin, Paul A. Jenkins, Gareth O. Roberts and Simon E.F. Spencer (2017). Simulation from quasi-stationary distributions on reducible state spaces. Advances in Applied Probability,Link opens in a new window 49 (3), 960-980.