Prof. James Kermode
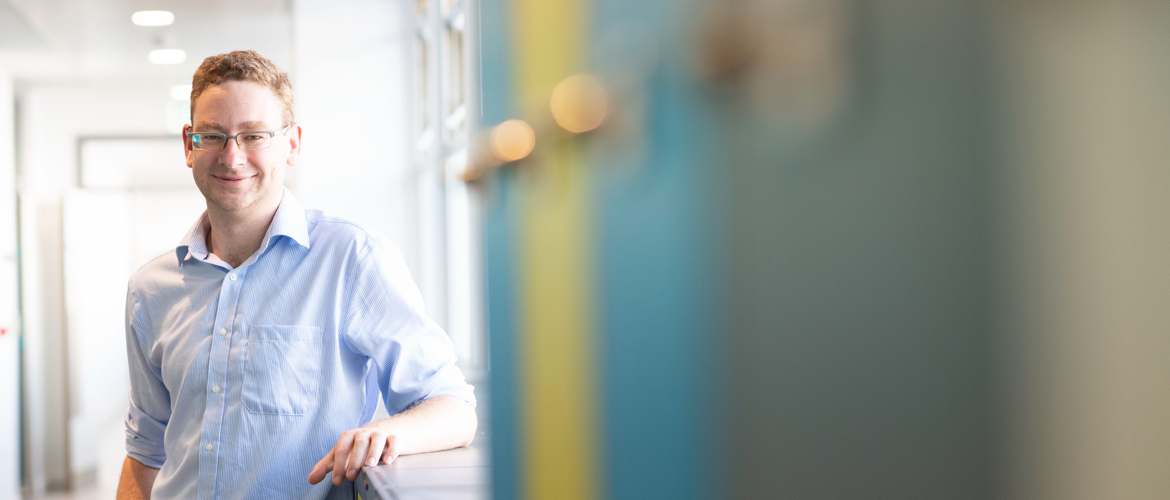
Prof. James Kermode
Professor of Materials Modelling
Head of Research and Deputy Head of Department
J.R.Kermode@warwick.ac.uk
+44 (0) 24 7652 8614
Biography
I am Professor of Materials Modelling in the School of Engineering at the University of Warwick, where I am also currently serving as Head of Research (Deputy Head of School). I also direct the EPSRC Centre for Doctoral Training in Modelling of Heterogeneous Systems (HetSys) and the Warwick Centre for Predictive Modelling (WCPM).
- Sept 2024-present Head of Research (Deputy Head of School), School of Engineering, University of Warwick
- Oct 2023-present Director, EPSRC Centre for Doctoral Training in Modelling of Heterogeneous Systems (HetSys)
- 2022-present Professor of Materials Modelling, School of Engineering, University of Warwick
- 2021-present Director, Warwick Centre for Predictive Modelling (WCPM)
- 2019-2022 Reader, School of Engineering, University of Warwick
- 2016-2019 Associate Professor, School of Engineering, University of Warwick
- 2014-2016 Assistant Professor, School of Engineering, University of Warwick
- 2009-2014 Postdoc in the Department of Physics at King's College London
- 2007-2008 Postdoc in the Department of Engineering at the University of Cambridge
- 2004-2007 PhD in the TCM Group at the Cavendish Laboratory, University of Cambridge
Research Interests
I develop multiscale materials modelling algorithms and the software that implements them, with a particular focus on machine learning and data-driven approaches, and on quantifying the uncertainty in the output of electronic structure and atomistic models. I am also active in applying parameter-free modelling techniques to make quantitative predictions of "chemomechanical" materials failure processes where stress and chemistry are tightly coupled, e.g. near the tip of a propagating crack (left), where local bond-breaking chemistry is driven by long-range stress fields. Prominent examples include:
|
|
Teaching Interests
In 2024/25 I will be teaching PX914 - Predictive Modelling and Uncertainty Quantification in the HetSys CDT and ES98E - Scientific Machine Learning in the MSc in Predictive Modelling and Scientific Computing.
Selected Publications
- P. Grigorev, A. M. Goryaeva, M.-C. Marinica, J. R. Kermode, and T. D. Swinburne,
Calculation of Dislocation Binding to Helium-Vacancy Defects in Tungsten Using Hybrid Ab Initio-Machine Learning Methods, Acta Mater. 247 118734 (2023) [arXiv:2111.11262] - L. Zhang, B. Onat, G. Dusson, A. McSloy, G. Anand, R. J. Maurer, C. Ortner, and J. R. Kermode, Equivariant Analytical Mapping of First Principles Hamiltonians to Accurate and Transferable Materials Models, npj Comp. Mater. 8, 158 (2022) [arXiv:2111.13736]
- S. Makri, C. Ortner and J. R. Kermode, A preconditioning scheme for Minimum Energy Path finding methods, J. Chem. Phys 150, 094109 (2019) [arXiv:1810.02705]
- A. P. Bartók, J. R. Kermode, N. Bernstein, and G. Csányi, Machine Learning a General-Purpose Interatomic Potential for Silicon, Phys. Rev. X 8, 041048 (2018)
- G. Sernicola, T. Giovannini, P. Patel, J. R. Kermode, D. S. Balint, T. Ben Britton, and F. Giuliani, In situ stable crack growth at the micron scale, Nat. Commun. 8, 108 (2017)
- J. R. Kermode, A. Gleizer, G. Kovel, L. Pastewka, G. Csányi, D Sherman and A De Vita, Low speed crack propagation via kink formation and advance on the silicon (110) cleavage plane,
Phys. Rev. Lett, 115 135501 (2015) - Z. Li, J. R. Kermode, and A. De Vita, Molecular Dynamics with On-the-Fly Machine Learning of Quantum-Mechanical Forces, Phys. Rev. Lett. 114, 096405 (2015)
- A. Gleizer, G. Peralta, J. R. Kermode, A. De Vita and D. Sherman, Dissociative Chemisorption of O2 Inducing Stress Corrosion Cracking in Silicon Crystals. Phys. Rev. Lett. 112, 115501 (2014)
- J. R. Kermode, L. Ben-Bashat, F. Atrash, J.J. Cilliers, D. Sherman and A. De Vita, Macroscopic scattering of cracks initiated at single impurity atoms. Nat. Commun. 4, 2441 (2013)
- J. R. Kermode, T. Albaret, D. Sherman, N. Bernstein, P. Gumbsch, M. C. Payne, G. Csányi and A. De Vita,
Low speed fracture instabilities in a brittle crystal, Nature 455, 1224-1227 (2008)
See also my full Publications page, my Talks page, and my profiles on ORCID, Google Scholar and the Warwick Research Archive Portal. My PhD Thesis is available from the University of Cambridge's repository.
Projects and Grants
- Co-director (2019-2023) and then Director of EPSRC Centre for Doctoral Training in Modelling of Heterogenous Systems
- I am a member of the UK Car Parinello Consortium (UKCP), which has EPSRC funding (2023-2026) to provide access to the UK national supercomputer ARCHER2.
Previous Research Projects
- Co-I for EPSRC standard mode proposal Multiscale Simulation for Rarefied Gas Flow in Engineering Design, with D. Lockerby (Warwick), C. White (Glasgow), M. Borg (Edinburgh) and L. Gibelli (Edinburgh)
- European Database for Multiscale Modelling of Radiation Damage (ENTENTE) Horizon 2020 Euratom NFRP-2019-2020 call, in consortium lead by CIEMAT.
- NOMAD Centre of Excellence (Horizon 2020 INFRAEDI-2019-1 call), in consortium lead by FHI Berlin. Warwick Co-Is were A. Bartok-Partay and R. Maurer
-
PI for Royce Institute Materials Challenge Accelerator Programme Hydrogen diffusion and trapping in multi-principal component alloys. Co-Is J. Darby (PDRA), R. Maurer and A. Bartok-Partay
- PI for Leverhulme Trust Research Project Grant proposal The Nature of Interatomic Forces in Metallic Systems with Christoph Ortner.
- EPSRC grant Boundary Conditions for Atomistic Simulation of Material Defects with C. Ortner (PI, Warwick), R. Catlow (Co-I, UCL) and Researcher Co-Is J. Braun (Warwick) and A. Sokol (UCL).
- EPSRC grant Reducing Risk through Uncertainty Quantification for Past, Present and Future Generations of Nuclear Power Plant in collaboration with Manchester and Bristol
- Hydrogen Embrittlement of Steels - I was an external associate of this EPSRC Programme Grant involving Oxford, Cambridge, Imperial, King's and Sheffield.
- My EPSRC First Grant Predictive Modelling of the Fundamentals of Failure in Metals (Aug 2016 - July 2018) had the overarching aim of developing new models to enable continuum-scale modelling of failure processes, in particular crack growth, by incorporating pre-computed first-principles information. This funding supported postdoctoral researcher Dr Petr Grigorev.
- The Novel Materials Discovery (NoMaD) laboratory, funded under the Horizon 2020 Centre of Excellence initiative, enables access to the huge amount of data routinely produced by computational materials science calculations through the online NoMaD repository. I was a senior researcher within the project, which is led by the Fritz Haber Institute and involves a consortium of 11 organisations throughout Europe. This project supported postdoctoral reseaarcher Dr Berk Onat.
- I held a Royal Society Research Project Grant (April 2017-Mar 2018) Bridging from Quantum Mechanical to Continuum Mechanical Models of Fracture, which provided funding for local computational resources.
- ARCHER eCSE project 11-7 (Sept 2017 - Feb 2018) supported postdoc Dr Letif Mones to implement preconditioned geometry optimisers in the CASTEP and ONETEP codes. Co-investigators were Dr Nick Hine and Prof. Christoph Ortner (Warwick) and Prof. Matt Probert (York).
- SiO2 Fracture: Chemomechanics with a Machine-Learning Hybrid QM/MM Scheme - project allocated 126 million core hours in 2016 on the Mira machine at the Argonne Leadership Computer Facility under the US DoE's INCITE programme to investigate stress corrosion cracking in silica. Co-investigators were Anatole von Lilienfeld and Alessandro De Vita.
- Multiscale Atomistic Simulation of the Mechanical Behaviour of Nickel-based Superalloys. Project allocated 20 million core hours at the Cineca and Jülich supercomputer centres. Other project members were Federico Bianchini, Alessio Comisso and Alessandro De Vita (KCL).
Vacancies
No vacancies at present. HetSys CDT PhD projects for Oct 25 start will be advertised in late autumn 2024.
Software
- I’m one of the authors of the libAtoms/QUIP molecular dynamics framework
- matscipy, a Python software library for computational materials science developed with Lars Pastewka.
- f90wrap, a utility for wrapping Fortran 95 code to make it accessible from Python, including support for derived types.
- Other software is available from my GitHub page, including an enhanced version of the AtomEye atomistic visualisation software which can read Extended XYZ (.xyz) and NetCDF (.nc) files (Note: the extended XYZ format is now also supported directly by OVITO and ASE).
External Collaborators
- Noam Bernstein (Naval Research Laboratory, Washington DC, USA)
- Maciej Buze (Birmingham, UK)
- Gábor Csányi (Cambridge, UK)
- Peter Gumbsch (Fraunhofer IWM, Germany)
- Gianpietro Moras (Fraunhofer IWM, Germany)
- Christoph Ortner (UBC, Vancouver)
- Lars Pastewka (Freiburg, Germany)
- Mike Payne (Cambridge, UK)
- Dov Sherman (Tel Aviv, Israel)
- Tom Swinburne (CiNAM Marseilles, France)