Content Blocks
10
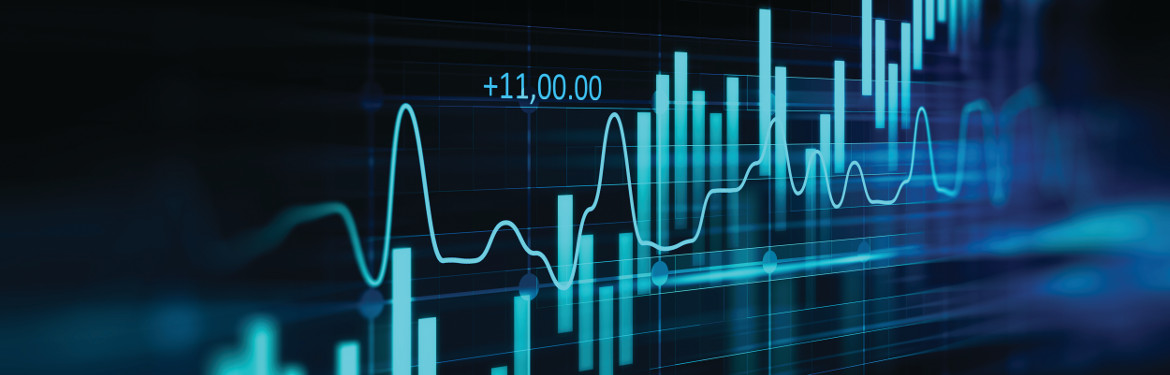
2a
P-L990 (MSc);
P-L991 (PGDip)
2b
MSc/PGDip
2c
MSc: 1 year full-time, 2 years part-time; PGDip: 9 months full-time, 18 months part-time
2d
2 October 2023
2e
2f
University of Warwick
3a
Join Warwick's Big Data and Digital Futures MSc/PGDip and learn to critically engage with big data. The Centre for Interdisciplinary Methodologies works across disciplines, drawing from the Arts, Humanities, Social Sciences and Sciences, to answer employers' demands for a new generation of researchers.
3b
This degree responds directly to the growing demand across research fields and by employers in society for a new generation of postgraduates who can critically engage with big data theoretically, methodologically and practically. In contrast to many big data-focused degrees (such as Data Science or Data Analytics) where the emphasis is almost exclusively on data practices and computational tools, this degree underpins key practical skills with a range of theoretical approaches to data.
How is our world influenced by big data? How are our lives represented in big data? This course will enable you, whatever your disciplinary background, to understand and act in a society transformed by data, networks and computation and develop a range of interdisciplinary capacities.
Our course offers you:
- Core knowledge in statistical modelling and programming for data-driven careers
- An extensive understanding of the relationship between big data technology and society
- Practical and critical application of these techniques to cutting-edge methods across the data spectrum
- R programming skills (using RStudio)
- Statistics in Social Science (up to multiple linear regression and logistic regression)
- Advanced Statistics (generalised linear models, multilevel modelling and casual inference)
- Basics in Social Network Analysis, Web Scraping, Reproducible Analysis, Data Visualisation, SQL, Deep Learning, Agent-Based Modelling (From Q-Step Masterclasses)
- Writing and communication skills for analysis/discussing technical content
- Critical academic research skills with an interdisciplinary focus
3d
Modules in this course make use of a range of teaching and learning techniques, including, for example:
- Blended learning including the use of an online virtual learning environment
- Student group and project work
- Lectures
- Seminars
- Reading and directed critical discussion
- Independent research by students
- Practice-based activities
3e
For this course a typical seminar size is around 16 students.
3f
There are around 7-9 hours contact hours per week for this course, depending on optional modules chosen.
3g
A combination of essays, reports, design projects, technical report writing, practice assessments, group work and presentations and an individual research project (10,000 word dissertation).
Reading lists
Most departments have reading lists available through Warwick Library. If you would like to view reading lists for the current cohort of students you can visit our Warwick Library web pageLink opens in a new window.
Your timetable
Your personalised timetable will be complete when you are registered for all modules, compulsory and optional, and you have been allocated to your lectures, seminars and other small group classes. Your compulsory modules will be registered for you and you will be able to choose your optional modules shortly before joining us.
4a
2:i undergraduate degree (or equivalent).
4b
- Band B
- IELTS overall score of 7.0, minimum component scores of two at 6.0/6.5 and the rest at 7.0 or above.
4c
There are no additional entry requirements for this course.
5a
Big Data Research: Hype or Revolution?
Big data is said to be transforming science and social science. In this module, you will critically engage with this claim and explore the ways in which the rapid rise of big data impacts on research processes and practices in a growing range of disciplinary areas and fields of study.
In particular, the module considers the following questions: What is big data? To what extent is 'big data' different to other kinds of data? What key issues are raised by big data? To what extent is big data transforming research practices? How are the 'nuts and bolts' of research practice (e.g. ethics, sampling, method, analysis, etc.) transformed with big data? To what extent are core concepts relating to research practice - such as comparison, description, explanation and prediction - transformed? To what extent can we critically engage with big data? How is big data transforming the 'discipline'?
Dissertation
The CIM Master’s dissertation is a piece of work (10,000 words) which addresses a single student-selected subject. The topic may concern any aspect of the subject matter of their Master’s programme. The dissertation is an exercise in independent study in which you can pursue a topic of interest. It allows you to further develop a range of independent research skills, including literature search and bibliography construction, theoretical argument, and generation/appraisal of empirical evidence.
Optional Core Modules
Term One
Data Science Across Disciplines
This module introduces students to the fundamental techniques, concepts and contemporary discussions across the broad field of data science. With data and data related artefacts becoming ubiquitous in all aspects of social life, data science gains access to new sources of data, is taken up across an expanding range of research fields and disciplines, and increasingly engages with societal challenges. The module provides an advanced introduction to the theoretical and scientific frameworks of data science, and to the fundamental techniques for working with data using appropriate procedures, algorithms and visualisation. Students learn how to critically approach data and data-driven artefacts, and engage with and critically reflect on contemporary discussions around the practice of data science, its compatibility with different analytics frameworks and disciplinary, and its relation to on-going digital transformations of society. As well as lectures discussing the theoretical, scientific and ethical frameworks of data science, the module features coding labs and workshops that expose students to the practice of working effectively with data, algorithms, and analytical techniques.
Or
Fundamentals in Quantitative Research Methods
This module has two aims: introduce to academic quantitative literature, secondary data acquisition and management, and the use of applied statistics in the social sciences; prepare to attend further statistical training (including PO92Q: Advanced Quantitative Research) and make use of statistics in future research works, such as master's or PhD dissertations.
This will not be an abstract statistics module, but a comprehensive approach to social and political numbers, in keeping with all method modules students may have attended previously and concurrently. It will include example data from diverse fields of social sciences, in particular surveys on attitudes and opinions. Exercises and essays will be based on a selection of datasets, such as the British Social Attitudes Survey, the European Social Survey, the World Values Survey, and the International Social Survey Programme.
Term Two
Scaling Data and Societies
Big data technologies involve scaling-up — scaling up quantities of data, scaling up data infrastructures, scaling up data management, and scaling up the number of participants in a given technological system. This module provides an understanding of the technical. methodological and conceptual changes in the new forms of thinking, research and engineering required for understanding and working with scalable socio-technical systems. Beginning with the question of what 'scale' is in general and how data-based transformations redefine the limits of scale, the module presents students with a series of different ‘lenses’ through which the impact of scale manifests itself differently across contemporary data spaces, including hands-on laboratory exercises. By the end of the module, students will have gained knowledge and a greater appreciation of the impact of big data on research in socio-technical systems at various scales and, conversely, the multiple ways in which the concept of scale is driving developments in big data.
Or
Advanced Quantitative Research
This module introduces students to a selected set of advanced statistical methods that are commonly used in quantitative social research. You will cover three advanced methods such as regression diagnostics and interactions, logistic and multinomial regression modelling, multilevel modelling, cluster analysis and factor analysis. These methods allow you to answer questions such as: Why do some people support a given public policy (e.g. the death penalty, Brexit or the GAFA tax), and others not? What are the main nuances and cleavages within a party (e.g. the Greens) or an ideological orientation (e.g. the populists)?
To gain hands-on experience with answering these questions with social and political science data of varying complexity, you will apply these methods to existing small- and large-scale data sets. The expectation is that by the end of the module you will understand the basic principles of the advanced statistical methods covered, appreciate the context in which the methods are best applied, and have had practical experience of applying these methods to real-world data.
5b
- Interdisciplinary Approaches to Machine Learning
- User Interface Cultures: Design, Method and Critique
- Visualisation Foundations
- Digital Sociology
- Complexity in the Social Sciences
- Digital Objects, Digital Methods
- Data Visualisation in Science, Culture and Public Policy
(8)
We have revised the information on this page since publication. See the edits we have made and content history.
This is a holding content block which does not currently display on the page. To make it live, update the copy above, change the Title to remove the brackets, and delete this sentence.