Systems Biology of Arabidopsis leaf senescence
We are using modeling techniques based on highly replicated timecourse microarray data to predict nodal regulatory genes in pathways leading to leaf senescence. Several highly replicated and detailed time course experiments have been carried out at Warwick HRI in collaboration with Warwick Systems Biology Centre to develop and test the network modelling methodology. Here we highlight one example in which the same leaf (leaf 7) was harvested from Arabidopsis plants at different times during development. The plants were growing in long days, 22 time points were harvested including a morning and afternoon sample on each day. Individual leaves were treated as biological replicates (no pooling was carried out to avoid data averaging). RNA was isolated from 4 individual leaves at each time point and four technical replicates probed to CATMA microarrays (31K genes) (176 arrays, 352 labelled samples). We have also carried out a similar analysis based on leaves isolated from plant growing in short days. Senescence in these leaves is likely to be under different regulation than that shown in leaves from plants grown in long days.
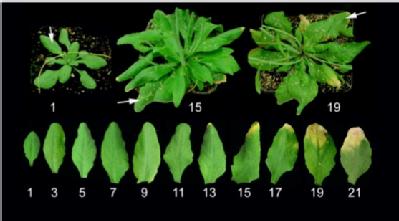
Development of Arabidopsis plants during the experiment showing the harvested leaf 7 at the morning harvest
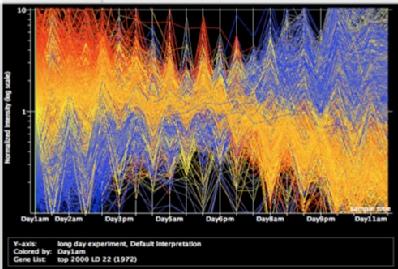
This graph shows the change in expression over the 22 timepoints for the 2000 most differentially expressed genes. Cluster analysis of these showed over 100 clusters showing different expression patterns.
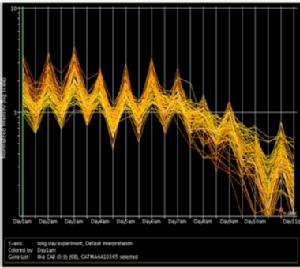
This graph shows one of these clusters enriched for Chlorophyll a/b binding protein genes.
State space models will be used to confirm and extend prior knowledge
We selected a small number of genes from clusters, from the developmental time course experiment. These included some genes for which we had previous biological data. State space modelling was undertaken to infer regulatory network models. The figure below shows a network model generated from the time course data. Arrows represent regulatory influences over consecutive time points. This model is exciting as it places a NAC domain transcription factor gene in a key hub position. We already know, from knock out and overexpression experiments with this gene, that this NAC gene has an important role in controlling at least some of the genes identified in this model including ZAT12, RAV2 and RD26, all of which are transcription factors shown to be important in stress response pathways. We have, therefore, demonstrated that this approach can be applied to the highly replicated time course array data to successfully generate networks that confirm prior knowledge. This network also immediately suggests many other novel linkages and regulatory connections all of which could be targets for experiments aimed at biological validation of the network.
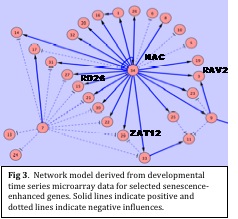
These baseline developmental datasets provide the foundations for assessing the extent of changes wrought by environmental stress which is the basis for the recently funded BBSRC SABR project Elucidating signalling networks in plant stress responses. Linking the models developed in data sets from a variety of different stresses with the developmental datasets described above will enable us to find the key genes involved in cross talk between the different stress responses and dentify candidates for future manipulation for improved stress resistance.