An introduction to Global Bias in RNA-seq data
A major goal of RNA-seq is the quantification of transcript abundances.
To this end, the number of sequencing reads mapping to a specific transcript is usually divided by its length, based on the assumption that length and expected read numbers are proportional. Such a linear model is embodied by the widely used TPM and FKPM measures.
Multiple local biases change the effective lengths of transcripts that can serve as sources for reads. While these biases are addressed by various software tools, a linear model is still used as the underlying assumption in all cases.
However, it has been shown that RNA-seq libraries are also affected by global biases that are specific to the protocol that was used for sample preparation, and which are potentially orders of magnitudes stronger than local biases. They cause a non-linear scaling between transcript length and expected read numbers.
This global bias can be visualized in the form of heatmaps that show sequencing read densities along transcripts (which are ordered from shorted to longest and aligned at 5' and 3' ends). The global bias appears as a global pattern that is characteristic for the used library preparation protocol (see figure below for different protocols).
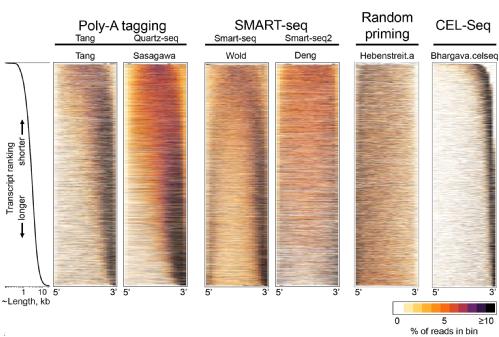
LiBiNorm uses these global read distributions from RNA-seq datasets to estimate parameters that are associated with models of the global biases that arise with different protocols. It uses this information to generate a bias-corrected, TPM-like measure of transcript abundance. The parameters can further be used to gain insights into the library preparation process which can be used in turn to optimized it.