Data Science MSci (UCAS G304)
Find out more about our Data Science MSci at Warwick
Our Data Science (MSci) degree provides an essential mix of highly developed mathematical, statistical and computing skills for those interested in working at the forefront of the modern data revolution. Our graduates are appropriately equipped to lead careers which leverage advanced technology to extract value from data or which develop such technologies.
General entry requirements
A level typical offer
For applicants taking Further Mathematics:
A*A*A to include A* A* in Mathematics and Further Mathematics
Or
A*AA to include A* A (in any order) in Mathematics and Further Mathematics and one of the following:
- STEP (grade 2)
- TMUA (score 5.0)
- AEA (Distinction)
Or
A*A*A*A to include A* A (in any order) in Mathematics and Further Mathematics
For applicants taking neither A level nor AS level Further Mathematics:
A*A*A* to include A* in Mathematics
Or
A*AA to include A* in Mathematics and one of the following:
- STEP (grade 2)
- TMUA (score 5.0)
- AEA (Distinction)
Please note, this course may assume knowledge from A level Further Mathematics. We advise that you undertake independent study, using for example the Advanced Mathematics Support Programme (AMSP), before starting your course.
A level contextual offer
We welcome applications from candidates who meet the contextual eligibility criteria. The typical contextual offer is
A*A*B, including A* in Mathematics and A* in Further Mathematics
Or
A*AB including A*, A in Mathematics and Further Mathematics (any order), plus grade 2 in any STEP/5.0 in TMUA/Distinction in AEA. See if you’re eligible.
If you are not taking Further Mathematics, the typical contextual offer is
A*A*A in three A levels to include A* in Mathematics
Or
A*AB to include A* in Maths plus either grade 2 in any STEP/5.0 in TMUA/Distinction in AEA.
General GCSE requirements
This section only applies to those who has studied GCSE as part of their curriculum. For those whose school curriculum did not include taking the GCSEs, please see the section below.
You will also need a minimum of GCSE grade 4 or C (or an equivalent qualification) in English Language. Find out more about our entry requirements and the qualifications we accept. We advise that you also check the English Language requirements for your course which may specify a higher GCSE English requirement. Please find the information about this below.
IB typical offer
39 overall to include 7 in Higher Level Mathematics 'Analysis and Approaches'
Or
38 overall to include 6 in Higher Level Mathematics 'Analysis and Approaches' and one of the following:
- STEP (grade 2)
- TMUA (score 5.0)
- AEA (Distinction)
Or
39 overall to include 7 in Higher Level Mathematics 'Applications and Interpretations'
Or
38 overall to include 6 in Higher Level Mathematics 'Applications and Interpretations' and one of the following:
- STEP (grade 2)
- TMUA (score 5.0)
- AEA (Distinction)
IB contextual offer
We welcome applications from candidates who meet the contextual eligibility criteria. The typical contextual offer is 37, including 7 in Higher Level Mathematics ‘Analysis and Approaches’ or 'Applications and Interpretations') or 36 overall including 6 in Higher Level Mathematics ‘Analysis and Approaches’ or 'Applications and Interpretations'), plus 2 in any STEP/5.0 in TMUA /Distinction in AEA. See if you’re eligible.
General GCSE requirements
This section only applies to those who has studied GCSE as part of their curriculum. For those whose school curriculum did not include taking the GCSEs, please see the section below.
You will also need a minimum of GCSE grade 4 or C (or an equivalent qualification) in English Language. Find out more about our entry requirements and the qualifications we accept. We advise that you also check the English Language requirements for your course which may specify a higher GCSE English requirement. Please find the information about this below.
BTEC
Level 3 BTECs will be considered alongside two A Levels including A Level Mathematics.
Scotland Advanced Highers
A1 in Advanced Higher Mathematics, A in one additional subject at Advanced Higher, and AAA in three additional Highers subjects.
Welsh Baccalaureate
A* in A level Mathematics, A* in A level Further Mathematics, B in a third A level, and C in the Advanced Skills Baccalaureate Wales
Or
A*, A in A level Mathematics and Further Mathematics (in any order), B in a third A level, C in the Advanced Skills Baccalaureate Wales, and 2 in STEP/5.0 in TMUA/ 2 in STEP/5.0 in TMUA/Distinction in AEA.
Access to Higher Education Diplomas
We will consider applicants returning to study who are presenting a QAA-recognised Access to Higher Education Diploma on a case-by-case basis.
Typically, we require 45 Credits at Level 3, including Distinction in 33 Level 3 credits and Merit in 12 Level 3 Credits. We may also require subject specific credits or an A level to be studied alongside the Access to Higher Education Diploma to fulfil essential subject requirements.
General GCSE requirements
This section only applies to those who has studied GCSE as part of their curriculum. For those whose school curriculum did not include taking the GCSEs, please see the section below.
You will also need a minimum of GCSE grade 4 or C (or an equivalent qualification) in English Language. Find out more about our entry requirements and the qualifications we accept. We advise that you also check the English Language requirements for your course which may specify a higher GCSE English requirement. Please find the information about this below.
International qualifications
Additional information
Applicants who are retaking or currently in a gap year are welcome to apply. If the Further Mathematics grade was achieved two years or more before entering university, applicants may be required to take the Further Mathematics exams again in the current academic year.
Native language A levels subjects are accepted as one of the three A level subjects. Please contact stats.ug.admissions@warwick.ac.uk if you have any questions.
Subject-specific international qualification requirements
Please consult the Departmental websiteLink opens in a new window for advice.
English Language requirements
All applicants have to meet our English Language requirementsLink opens in a new window. If you cannot demonstrate that you meet these, you may be invited to take part in our Pre-sessional English course at WarwickLink opens in a new window.
This course requires: Band B
Learn more about our English Language requirementsLink opens in a new window.
Frequently asked questions
Warwick may make differential offers to students in a number of circumstances. These include students participating in a Widening Participation programme or who meet the contextual data criteria.
Differential offers will usually be one or two grades below Warwick’s standard offer.
All students who successfully complete the Warwick IFP and apply to Warwick through UCAS will receive a guaranteed conditional offer for a related undergraduate programme (selected courses only).
Find out more about standard offers and conditions for the IFP.
We welcome applications for deferred entry.
We do not typically interview applicants. Offers are made based on your UCAS form which includes predicted and actual grades, your personal statement and school reference.
Course overview
Data Science questions how to make sense of the vast volumes of data generated daily in modern life, from social networks to scientific research and finance. It then suggests sophisticated computing techniques for processing this deluge of information.
The degree in Data Science provides an essential mix of highly developed mathematical, statistical and computing skills for those interested in working at the forefront of the modern data revolution, that is, in a career which leverages advanced technology to extract value from data - or in developing such technology.
Taught by specialists from the departments of Statistics, Computer Science and Mathematics, you will develop expertise in specialist areas of machine learning, data mining and algorithmic complexity. Skills development in mathematical and statistical modelling, algorithm design and software engineering prepares you for other careers including manufacturing, pharmaceuticals, finance, telecoms and scientific research. The BSc and MSci in Data Science are the same during the first two years, making it easy to reconsider your preference.
The fourth year of the MSci degree offers a supervised Master's level project and the possibility to specialise in areas such as advanced statistical learning and big data, high-performance computing, algorithmic game theory, and computational biology and statistical genetics.
Actuarial Exemptions
Information about possible exemptions for professional actuarial examinations for students on this course can be found in the Departmental webpageLink opens in a new windowLink opens in a new window.
Study abroad
We support student mobility through study abroad programmes and all students have the opportunity to apply for an intercalated year abroad at one of our partner universities. The Study Abroad Team based in the Office for Student Opportunity offers support for these activities, and the Department's dedicated Study Abroad Co-ordinator can provide more specific information and assistance.
Core modules
You will learn through a combination of lectures, small-group tutorials and practical sessions based in the Department's well-equipped undergraduate computing laboratory. A central part of learning in Data Science is problem solving.
The curriculum is built on the principle that module choices get more and more flexible as you progress through the degree. On top of that, you may choose to study additional options from an even wider range of modules.
- Year One: 100% core
- Year Two: about 20% optional modules
- Year Three: about 60% optional modules
- Year Four: about 75% optional modules
Year One
Refresher Mathematics
This is a pre-sessional course for incoming first-year undergraduates from mathematics and joint-mathematics courses. The aim is to refresh A Level mathematics and certain core items from further mathematics in preparation for starting their degree.
Read more about the Refresher Mathematics module,Link opens in a new window including the methods of teaching and assessment (content applies to 2024/25 year of study).
Programming for Computer Scientists
This module aims to help you develop your programming skills, regardless of your starting skill level. You will develop problem solving skills through the lens of procedural and object-oriented programming. Using the Java programming language, you will engage with practical work that shall enable you to learn concepts such as classes, encapsulations, arrays, inheritance and advanced topics such as multi-threading and reflection. By engaging with the Warwick Robot Maze environment, you can expect to gain skills in errors analysis and debugging that will help you produce well-designed and well-tested code.
Read more about the Programming for Computer Scientists moduleLink opens in a new window, including the methods of teaching and assessment (content applies to 2024/25 year of study).
Design of Information Structures
Following on from Programming for Computer Scientists, on the fundamentals of programming, this module will teach you all about data structures and how to program them. We will look at how we can represent data structures efficiently and how we can apply formal reasoning to them. You will also study algorithms that use data structures. Successful completion will see you able to understand the structures and concepts underpinning object-oriented programming, and able to write programs that operate on large data sets.
Read more about the Design of Information Structures moduleLink opens in a new window, including the methods of teaching and assessment (content applies to 2024/25 year of study).
Mathematical Programming I
Operational Research is concerned with advanced analytical methods to support decision making, for example for resource allocation, routing or scheduling. A common problem in decision making is finding an optimal solution subject to certain constraints. Mathematical Programming I introduces you to theoretical and practical aspects of linear programming, a mathematical approach to such optimisation problems.
Read more about the Mathematical Programming I moduleLink opens in a new window, including the methods of teaching and assessment (content applies to 2022/23 year of study).
Vectors and Matrices
Many problems in maths and science are solved by reduction to a system of simultaneous linear equations in a number of variables. Even for problems which cannot be solved in this way, it is often possible to obtain an approximate solution by solving a system of simultaneous linear equations, giving the "best possible linear approximation''.
The branch of maths treating simultaneous linear equations is called linear algebra. The module contains a theoretical algebraic core, whose main idea is that of a vector space and of a linear map from one vector space to another. It discusses the concepts of a basis in a vector space, the dimension of a vector space, the image and kernel of a linear map, the rank and nullity of a linear map, and the representation of a linear map by means of a matrix.
These theoretical ideas have many applications, which will be discussed in the module. These applications include:
Solutions of simultaneous linear equations. Properties of vectors. Properties of matrices, such as rank, row reduction, eigenvalues and eigenvectors. Properties of determinants and ways of calculating them.
Read more about the Vectors and Matrices moduleLink opens in a new window, including the methods of teaching and assessment (content applies to 2024/25 year of study).
Calculus 1/2
Calculus is the mathematical study of continuous change. In this module there will be considerable emphasis throughout on the need to argue with much greater precision and care than you had to at school. With the support of your fellow students, lecturers and other helpers, you will be encouraged to move on from the situation where the teacher shows you how to solve each kind of problem, to the point where you can develop your own methods for solving problems. By the end of the year you will be able to answer interesting questions like, what do we mean by `infinity’?
Read more about these modules, including the methods of teaching and assessment (content applies to 2024/25 year of study):
Sets and Numbers
Mathematics can be described as the science of logical deduction - if we assume such and such as given, what can we deduce with absolute certainty? Consequently, mathematics has a very high standard of truth - the only way to establish a mathematical claim is to give a complete, rigorous proof. Sets and Numbers aims to show students what can be achieved through abstract mathematical reasoning.
Read more about the Sets and Numbers moduleLink opens in a new window, including the methods of teaching and assessment (content applies to 2024/25 year of study).
Introduction to Statistical Modelling
This module is an introduction to statistical thinking and inference. You’ll learn how the concepts you met from Probability can be used to construct a statistical model – a coherent explanation for data. You’ll be able to propose appropriate models for some simple datasets, and along the way you’ll discover how a function called the likelihood plays a key role in the foundations of statistical inference. You will also be introduced to the fundamental ideas of regression. Using the R software package you’ll become familiar with the statistical analysis pipeline: exploratory data analysis, formulating a model, assessing its fit, and visualising and communicating results. The module also prepares you for a more in-depth look at Mathematical Statistics in Year Two.
Read more about the Introduction to Statistical Modelling moduleLink opens in a new window, including the methods of teaching and assessment (content applies to 2024/25 year of study).
Probability 1
Probability is a foundational module that will introduce you both to the important concepts in probability but also the key notions of mathematical formalism and problem-solving. Want to think like a mathematician? This module is for you. You will learn how to express mathematical concepts clearly and precisely and how to construct rigorous mathematical arguments through examples from probability, enhancing your mathematical and logical reasoning skills. You will also develop your ability to calculate using probabilities and expectations by experimenting with random outcomes through the notion of events and their probability. You’ll learn counting methods (inclusion–exclusion formula and binomial co-efficients), and study theoretical topics including conditional probability and Bayes’ Theorem.
Read more about the Probability 1 moduleLink opens in a new window, including the methods of teaching and assessment (content applies to 2024/25 year of study).
Probability 2
This module continues from Probability I, which prepares you to investigate probability theory in further detail here. Now you will look at examples of both discrete and continuous probability spaces. You’ll scrutinise important families of distributions and the distribution of random variables, and the light this shines on the properties of expectation. You’ll examine mean, variance and co-variance of distribution, through Chebyshev's and Cauchy-Schwarz inequalities, as well as the concept of conditional expectation. The module provides important grounding for later study in advanced probability, statistical modelling, and other areas of potential specialisation such as mathematical finance.
Read more about the Probability 2 moduleLink opens in a new window, including the methods of teaching and assessment (content applies to 2024/25 year of study).
Year Two
Software Engineering
Centred on teamwork, you will concentrate on applying software engineering principles to develop a significant software system with your peers from feasibility studies through modelling, design, implementation, evaluation, maintenance and evolution. You’ll focus on design quality, human–computer interaction, technical evaluation, teamwork and project management. With a deeper appreciation of the stages of the software life-cycle, you’ll gain skills to design object-oriented software using formal modelling and notation. You will be taught the principles of graphical user interface and user-centred design, and be able to evaluate projects in the light of factors ranging from technical accomplishment and project management, to communication and successful teamwork.
Read more about the Software Engineering moduleLink opens in a new window, including the methods of teaching and assessment (content applies to 2024/25 year of study).
Database Systems
During this module, you will learn how relational database theory can be used to efficiently organise and retrieve large amounts of data. This includes a study of different relational query languages and practical experience of the SQL language that is widely used in industry. Successful completion will see you equipped to create appropriate, efficient database designs for a range of applications and to translate informal queries into formal notation. You will have learned to identify appropriate data constraints to ensure the integrity of the database and to mitigate various common security threats.
Read more about the Database Systems moduleLink opens in a new window, including the methods of teaching and assessment (content applies to 2024/25 year of study).
Algorithms
Data structures and algorithms are fundamental to programming and to understanding computation. In this module, you will be using sophisticated tools to apply algorithmic techniques to computational problems. By the close of the course, you’ll have studied a variety of data structures and will be using them for the design and implementation of algorithms, including testing and proofing, and analysing their efficiency. This is a practical course, so expect to be working on real-life problems using elementary graph, greedy, and divide-and-conquer algorithms, as well as gaining knowledge on dynamic programming and network flows.
Read more about the Algorithms moduleLink opens in a new window, including the methods of teaching and assessment (content applies to 2024/25 year of study).
Stochastic Processes
The concept of a stochastic (developing randomly over time) process is a useful and surprisingly beautiful mathematical tool in economics, biology, psychology and operations research. In studying the ideas governing stochastic processes, you’ll learn in detail about random walks – the building blocks for constructing other processes as well as being important in their own right, and a special kind of ‘memoryless’ stochastic process known as a Markov chain, which has an enormous range of application and a large and beautiful underlying theory. Your understanding will extend to notions of behaviour, including transience, recurrence and equilibrium, and you will apply these ideas to problems in probability theory.
Read more about the Stochastic Processes moduleLink opens in a new window, including the methods of teaching and assessment (content applies to 2024/25 year of study).
Mathematical Methods for Statistics and Probability
Following the mathematical modules in Year One, you’ll gain expertise in the application of mathematical techniques to probability and statistics. For example, you’ll be able to adapt the techniques of calculus to compute expectations and conditional distributions relating to a random vector, and you’ll encounter the matrix theory needed to understand covariance structure. You’ll also gain a grounding in the linear algebra underlying regression (such as inner product spaces and orthogonalization). By the end of your course, expect to apply multivariate calculus (integration, calculation of under-surface volumes, variable formulae and Fubini’s Theorem), to use partial derivatives, to derive critical points and extrema, and to understand constrained optimisation. You’ll also work on eigenvalues and eigenvectors, diagonalisation, orthogonal bases and orthonormalisation.
Read more about the Mathematical Methods for Statistics and Probability moduleLink opens in a new window, including the methods of teaching and assessment (content applies to 2024/25 year of study).
Probability for Mathematical Statistics
If you have already completed Probability in Year One, on this module you’ll have the opportunity to acquire the knowledge you need to study more advanced topics in probability and to understand the bridge between probability and statistics. You’ll study discrete, continuous and multivariate distributions in greater depth, and also learn about Jacobian transformation formula, conditional and multivariate Gaussian distributions, and the related distributions Chi-squared, Student’s and Fisher. You will also cover more advanced topics including moment-generating functions for random variables, notions of convergence, and the Law of Large Numbers and the Central Limit Theorem.
Read more about the Probability for Mathematical Statistics moduleLink opens in a new window, including the methods of teaching and assessment (content applies to 2024/25 year of study).
Mathematical Statistics
If you’ve completed “Probability for Mathematical Statistics”, this second-term module is your next step, where you’ll study in detail the major ideas behind statistical inference, with an emphasis on statistical modelling and likelihoods. You’ll learn how to estimate the parameters of a statistical model through the theory of estimators, and how to choose between competing explanations of your data through model selection. This leads you on to important concepts including hypothesis testing, p-values, and confidence intervals, ideas widely used across numerous scientific disciplines. You’ll also discover the ideas underlying Bayesian statistics, a flexible and intuitive approach to inference which is especially amenable to modern computational techniques. Overall this module will provide you a very firm foundation for your future engagement in advanced statistics – in your final years and beyond.
Read more about the Mathematical Statistics moduleLink opens in a new window, including the methods of teaching and assessment (content applies to 2024/25 year of study).
Linear Statistical Modelling with R
This module runs in parallel with Mathematical Statistics and gives you hands-on experience in using some of the ideas you saw there. The centrepiece of this module is the notion of a linear model, which allows you to formulate a regression model to explain the relationship between predictor variables and response variables. You will discover key ideas of regression (such as residuals, diagnostics, sampling distributions, least squares estimators, analysis of variance, t-tests and F-tests) and you will analyse estimators for a variety of regression problems. This module has a strong practical component and you will use the software package R to analyse datasets, including exploratory data analysis, fitting and assessing linear models, and communicating your results. The module will prepare you for numerous final years modules, notably the Year Three module covering the (even more flexible) generalised linear models.
Read more about the Linear Statistical Modelling with R moduleLink opens in a new window, including the methods of teaching and assessment (content applies to 2024/25 year of study).
Year Three
BSc and MSci in Data Science are the same during the first two years, making it easy to reconsider your preference. Differences become apparent in the final years. The third year of MSci involves a module whose aim is to prepare you for the statistical investigative cycle from problem formulation to the communication of conclusions. The fourth (final) year of MSci offers a range of advanced modules from across Data Science, and you also choose a Master's level dissertation project from a wide selection of topics.
Optional modules
Optional modules can vary from year to year. Example optional modules may include:
- Artificial Intelligence
- Games and Decisions
- Neural Computing
- Machine Learning
- Approximation and Randomised Algorithms
- Mobile Robotics
- Computer Graphics
- Professional Practice of Data Analysis
Assessment
You will be assessed by a combination of closed and open-book examinations, continuous assessment and project work, depending on your options. Your third year will contain a Data Science project and your fourth year will contain a research project.
The first year counts 10%, the second year 20%, the third year 30% and the fourth year 40% towards the final MSci degree mark.
Teaching
The curriculum is divided up into modules consisting of lectures and assessments, which are often supplemented by smaller group teaching such as tutorials, supervisions and computer labs. Homework assignments for each module are often biweekly and the expectation is that students work hard trying to tackle problems covering a range of levels of difficulty.
Class sizes
Class sizes vary from module to module. While selected optional modules may have smaller class sizes, some core modules may have over 300 students. Support classes for core modules usually consist of around 20 students.
Typical contact hours
Contact time is around 15 hours a week.
Tuition fees
Tuition fees cover the majority of the costs of your study, including teaching and assessment. Fees are charged at the start of each academic year. If you pay your fees directly to the University, you can choose to pay in instalments.
Undergraduate fees
On 4 November the UK government announced an increase in the tuition fee cap for Home students for academic year 2025/26. The University of Warwick’s Executive Board has consequently confirmed a change in fees from the previously advertised rate to £9,535.
The University expects to increase fees for future years in line with any inflationary uplift as determined by the UK government.
How are fees set?
The UK Government sets tuition fee rates.
To learn more about how the UK student fees and maintenance loans are set, please visit the UK Government websiteLink opens in a new window and UCASLink opens in a new window.
Undergraduate fees
If you are an overseas student enrolling in 2025-26, your annual tuition fees will be as follows:
- Band 1 – £26,290 per year (classroom-based courses, including Humanities and most Social Science courses)
- Band 2 – £33,520 per year (laboratory-based courses, plus Maths, Statistics, Theatre and Performance Studies, Economics, and courses provided by Warwick Business School, with exceptions)
Tuition fees for 2026 entry have not been set. We will publish updated information here as soon as it becomes available, so please check back for updates about 2026 fee rates before you apply.
Fee status guidance
We carry out an initial fee status assessment based on the information you provide in your application. Students will be classified as Home or Overseas fee status. Your fee status determines tuition fees, and what financial support and scholarships may be available. If you receive an offer, your fee status will be clearly stated alongside the tuition fee information.
Do you need your fee classification to be reviewed?
If you believe that your fee status has been classified incorrectly, you can complete a fee status assessment questionnaire. Please follow the instructions in your offer information and provide the documents needed to reassess your status.
Find out more about how universities assess fee status.Link opens in a new window
Additional course costs
As well as tuition fees and living expenses, some courses may require you to cover the cost of field trips or costs associated with travel abroad.
For departmental specific costs, please see the Modules tab on this web page for the list of core and optional core modules with hyperlinks to our Module CatalogueLink opens in a new window (please visit the Department’s website if the Module Catalogue hyperlinks are not provided).
Associated costs can be found on the Study tab for each module listed in the Module Catalogue (please note most of the module content applies to 2024/25 year of study). Information about module specific costs should be considered in conjunction with the more general costs below:
- Core text books
- Printer credits
- Dissertation binding
- Robe hire for your degree ceremony
Further information
Find out more about tuition fees from our Student Finance team.
Scholarships and bursaries
Learn about scholarships and bursaries available to undergraduate students.
We offer a number of undergraduate scholarships and bursaries to full-time undergraduate students. These include sporting and musical bursaries, and scholarships offered by commercial organisations.
Find out more about funding opportunities for full-time students.Link opens in a new window
If you are an international student, a limited number of scholarships may be available.
Find out more information on our international scholarship pages.Link opens in a new window
You may be eligible for financial help from your own government, from the British Council or from other funding agencies. You can usually request information on scholarships from the Ministry of Education in your home country, or from the local British Council office.
Warwick Undergraduate Global Excellence Scholarship
We believe there should be no barrier to talent. That's why we are committed to offering a scholarship that makes it easier for gifted, ambitious international learners to pursue their academic interests at one of the UK's most prestigious universities.
We provide extra financial support for qualifying students from lower income families. The Warwick Undergraduate Bursary is an annual award of up to £2,500 per annum. It is intended to help with course-related costs and you do not have to pay it back.
As part of the 'City of Sanctuary' movement, we are committed to building a culture of hospitality and welcome, especially for those seeking sanctuary from war and persecution. We provide a range of scholarships to enable people seeking sanctuary or asylum to progress to access university education.
Further information
Find out more about Warwick undergraduate bursaries and scholarships.
Eligibility for student loans
Your eligibility for student finance will depend on certain criteria, such as your nationality and residency status, your course, and previous study at higher education level.
Check if you're eligible for student finance.
Tuition Fee Loan
You can apply for a Tuition Fee Loan to cover your tuition fees. It is non-means tested, which means the amount you can receive is not based on your household income. The Loan is paid directly to the University so, if you choose to take the full Tuition Fee Loan, you won’t have to set up any payments.
Maintenance Loan for living costs
You can apply for a Maintenance Loan towards your living costs such as accommodation, food and bills. This loan is means-tested, so the amount you receive is partially based on your household income and whether you choose to live at home or in student accommodation.
If you’re starting a course on or after 1 August 2021, you usually must have settled or pre-settled status under the EU Settlement SchemeLink opens in a new window to get student finance.
Tuition Fee Loan
If you are an EU student and eligible for student finance you may be able to get a Tuition Fee Loan to cover your fees. It is non-means tested, which means the amount you may receive is not based on your household income. The Loan is paid directly to the University so, if you choose to take the full Tuition Fee Loan, you won't have to set up any payments.
Help with living costs
If you struggle to meet your essential living costs, our Student Funding team Link opens in a new windowwill be on hand to offer advice and support.
There are a number of options that may be available to you including government, bursary and/or hardship support. Warwick also has a number of bursaries and scholarships Link opens in a new windowthat you may be eligible to apply for.
Repaying your loans
You will repay your loan or loans gradually once you are working and earning above a certain amount. For students starting their course after 1 August 2023, the repayment threshold is £25,000. Repayments will be taken directly from your salary if you are an employee. If your income falls below the earnings threshold, your repayments will stop until your income goes back up above this figure.
Find out more about repaying your student loanLink opens in a new window.
Placements and work experience
You may additionally choose to spend an intercalated year in an approved industry, business or university between your last two years at Warwick, subject to availability and to satisfactory academic performance.
Your career
Recent graduates have pursued job roles such as:
- Actuaries, economists and statisticians
- Software developers
- Chartered and certified accountants
- Finance and investment analysts
- Teachers
- Telecommunication designers
- Data scientists and engineers
- Academics
UK firms that have employed recent Warwick graduates from the Mathematics and Statistics Departments include:
- Adder Technology
- Astra Zeneca
- Amazon
- BlackRock International
- Merrill Lynch
- Brainlabs
- Civil Service
- Conduent
- Darktrace
- Deloitte
- Department of Health
- eBay
- Ford Motor Company
- Fore Consulting
- Goldman Sachs
- Government Actuaries
- Investec
- Jane Street Capital
- KPMG
- Lloyds
- MBDA
- Metaswitch
- Met Office
- Ministry of Justice
- RenaissanceRe (Syndicate 1458)
- Oxford Clinical Trials Unit
- Softwire
- Solid Solutions
- Sword Apak
- Ten10
- Towers Watson
- Xafinity
Supporting your career
Our department has a dedicated professionally qualified Senior Careers Consultant to support you. They offer impartial advice and guidance, together with workshops and events throughout the year. Previous examples of workshops and events include:
- Finding experience to boost your CV in Year One and Two
- Careers options with a degree in Statistics
- Warwick careers fairs throughout the year
- Interview skills for Statistics students
- Maths and Stats Careers Fair
Find out more about careers support at Warwick.Link opens in a new window
Statistics at Warwick
Find out more about us on our websiteLink opens in a new window
Our courses
- Data Science (BSc)
- Data Science (MSci)
- Mathematics and Statistics (BSc)
- Mathematics and Statistics (MMathStat)
- MORSE (Mathematics, Operational Research, Statistics and Economics) (BSc)
- MMORSE (Mathematics, Operational Research, Statistics and Economics)
Related degrees
Life at Warwick
Within a close-knit community of staff and students from all over the world, discover a campus alive with possibilities. A place where all the elements of your student experience come together in one place. Our supportive, energising, welcoming space creates the ideal environment for forging new connections, having fun and finding inspiration.
Find out how to apply to us, ask your questions, and find out more.
Warwick Accommodation
Finding the right accommodation is key to helping you settle in quickly.
We have a range of residences for undergraduate students on campus.
Our campus
You won't be short of ways to spend your time on campus - whether it's visiting Warwick Arts Centre, using our incredible new sports facilities, socialising in our bars, nightclub and cafés, or enjoying an open-air event. Or if you need some peace and quiet, you can explore lakes, woodland and green spaces just a few minutes’ walk from central campus.
Food and drink
We have lots of cafés, restaurants and shops on campus. You can enjoy great quality food and drink, with plenty of choice for all tastes and budgets. There is a convenience store on central campus, as well as two supermarkets and a small shopping centre in the nearby Cannon Park Retail Park. Several of them offer delivery services to help you stay stocked up.
And don't miss our regular food market day on the Piazza with tempting, fresh and delicious street food. Soak up the atmosphere and try something new, with mouth-watering food for all tastes.
Clubs and societies
We currently have more than 300 student-run societies.
So whether you’re into films, martial arts, astronomy, gaming or musical theatre, you can instantly connect with people with similar interests.
Or you could try something new, or even form your own society.
Sports and fitness
Staying active at Warwick is no sweat, thanks to our amazing new Sports and Wellness Hub, indoor and outdoor tennis centre, 60 acres of sports pitches, and more than 60 sports clubs.
Whether you want to compete, relax or just have fun, you can achieve your fitness goals.
Studying on campus
Our campus is designed to cater for all of your learning needs.
You will benefit from a variety of flexible, well-equipped study spaces and teaching facilities across the University.
- The Oculus, our outstanding learning hub, houses state-of-the-art lecture theatres and innovative social learning and network areas.
- The University Library provides access to over one million printed works and tens of thousands of electronic journals
- Different study spaces offering you flexible individual and group study spaces.
Travel and local area
Our campus is in Coventry, a modern city with high street shops, restaurants, nightclubs and bars sitting alongside medieval monuments. The Warwickshire towns of Leamington Spa and Kenilworth are also nearby.
The University is close to major road, rail and air links. London is just an hour by direct train from Coventry, with Birmingham a 20-minute trip. Birmingham International Airport is nearby (a 20-minute drive).
Wellbeing support and faith provision
Our continuous support network is here to help you adjust to student life and to ensure you can easily access advice on many different issues. These may include managing your finances and workload, and settling into shared accommodation. We also have specialist disability and mental health support teams.
Our Chaplaincy is home to Chaplains from the Christian, Jewish and Muslim faiths. We provide regular services for all Christian denominations and a Shabbat meal every Friday for our Jewish students. There is also an Islamic prayer hall, halal kitchen and ablution facilities.
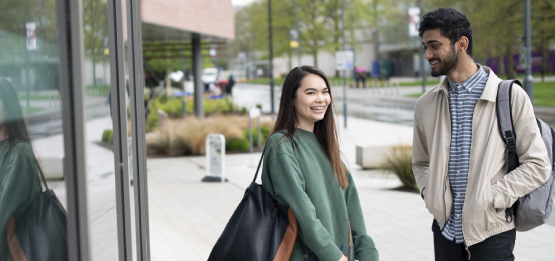
How to apply
Learn more about our application process.

Key dates
Key dates for your application to Warwick.
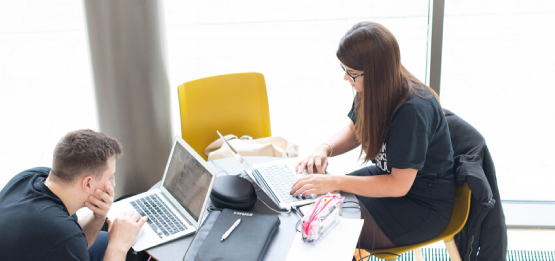
Writing your personal statement
Make an impression and demonstrate your passion for your course.
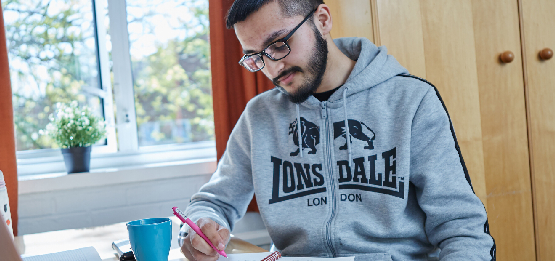
After you've applied
Find out how we process your application.
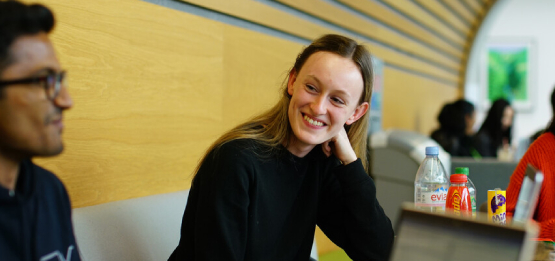
Our Admission Statement
Read Warwick's Admission Statement
3 ways to connect
Talk to us
Join us at a live event. You can ask about courses, applying to Warwick, life at Warwick, visas and immigration, and more.
Warwick Experience
Take a virtual, student-led campus tour. Then join an interactive panel session, where you can hear from and chat to our current students and staff.
Student blogs
Explore our student blogs in Unibuddy. You can read about campus life from students themselves, and register to post questions directly to students.
Explore campus with our virtual tour
Our 360 tour lets you:
- Watch student videos
- View 360 photography and drone footage
- Learn about facilities and landmarks
Explore our campus virtually through our 360 campus tour now
Come to an Open Day
Don’t just take it from us, come and see for yourself what Warwick is all about. Whether it's a virtual visit or in-person, our University Open Days give you the chance to meet staff and students, visit academic departments, tour the campus and get a real feel for life at Warwick.
Sign up for updates
Discover more about our courses and campus life with our helpful information and timely reminders.