Applied Microeconomics
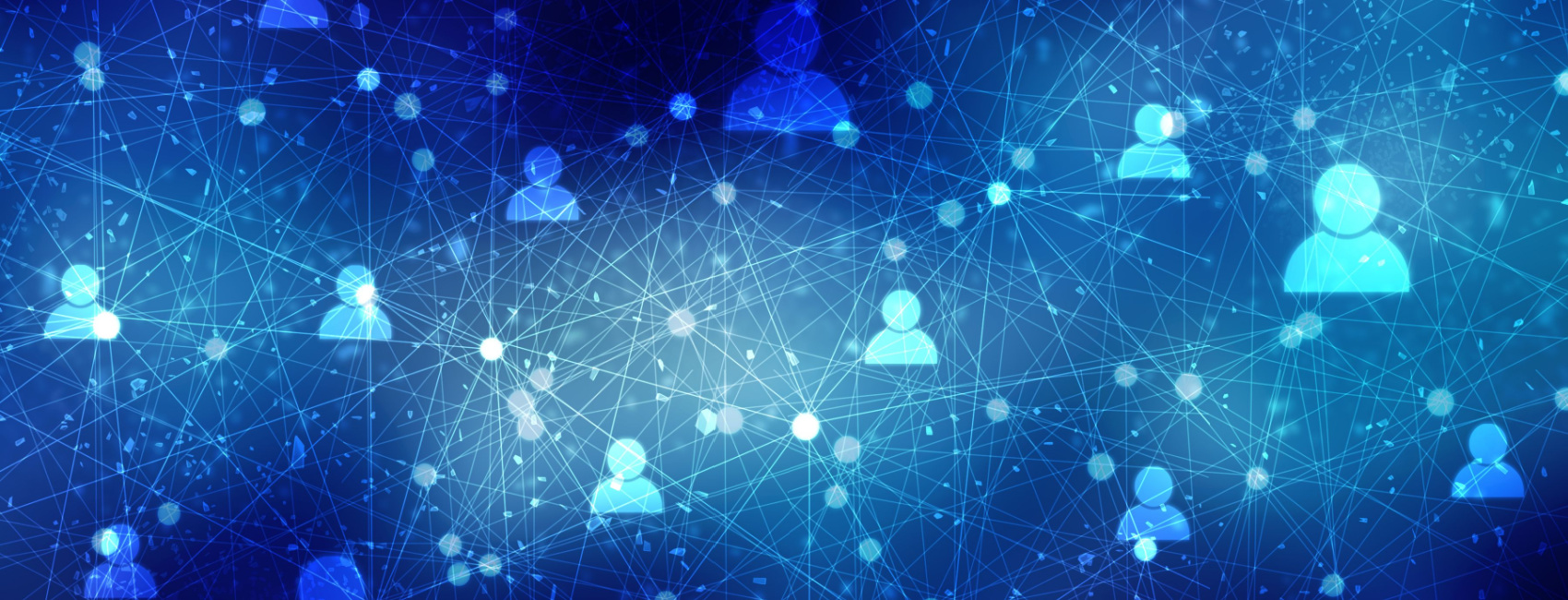
Applied Microeconomics
The Applied Microeconomics research group unites researchers working on a broad array of topics within such areas as labour economics, economics of education, health economics, family economics, urban economics, environmental economics, and the economics of science and innovation. The group operates in close collaboration with the CAGE Research Centre.
The group participates in the CAGE seminar on Applied Economics, which runs weekly on Tuesdays at 2:15pm. Students and faculty members of the group present their ongoing work in two brown bag seminars, held weekly on Tuesdays and Wednesdays at 1pm. Students, in collaboration with faculty members, also organise a bi-weekly reading group in applied econometrics on Thursdays at 1pm. The group organises numerous events throughout the year, including the Research Away Day and several thematic workshops.
Our activities
Work in Progress seminars
Tuesdays and Wednesdays 1-2pm
Students and faculty members of the group present their work in progress in two brown bag seminars. See below for a detailed scheduled of speakers.
Applied Econometrics reading group
Thursdays (bi-weekly) 1-2pm
Organised by students in collaboration with faculty members. See the Events calendar below for further details
People
Academics
Academics associated with the Applied Microeconomics Group are:
Research Students
Events
Econometrics Seminar - Bryan Graham (UC Berkeley)
Title: Simulated Maximum Likelihood Estimation of Large Games using Scenarios". This is joint work with Andrin Pelican.
Abstract: This paper introduces a new simulation algorithm for evaluating the
log-likelihood and score functions associated with a class of supermodular
complete information discrete games. The algorithm allows for payoff
function estimation in games with large numbers of players and/or
many binary actions per player (e.g., games with tens of thousands
of strategic binary actions). In such cases the likelihood of the
observed pure strategy combination may be (i) very small and (ii)
a high dimensional integral with a complex integration region. Direct
numerical integration, as well as accept-reject Monte Carlo integration,
are computationally impractical in such settings. In contrast, our
method allows for accurate likelihood simulation with modest numbers
of simulation draws. Use cases include simulated maximum likelihood
(SML) parameter estimation in models of technology adoption, peer
effects, and strategic network formation.