Applied Microeconomics
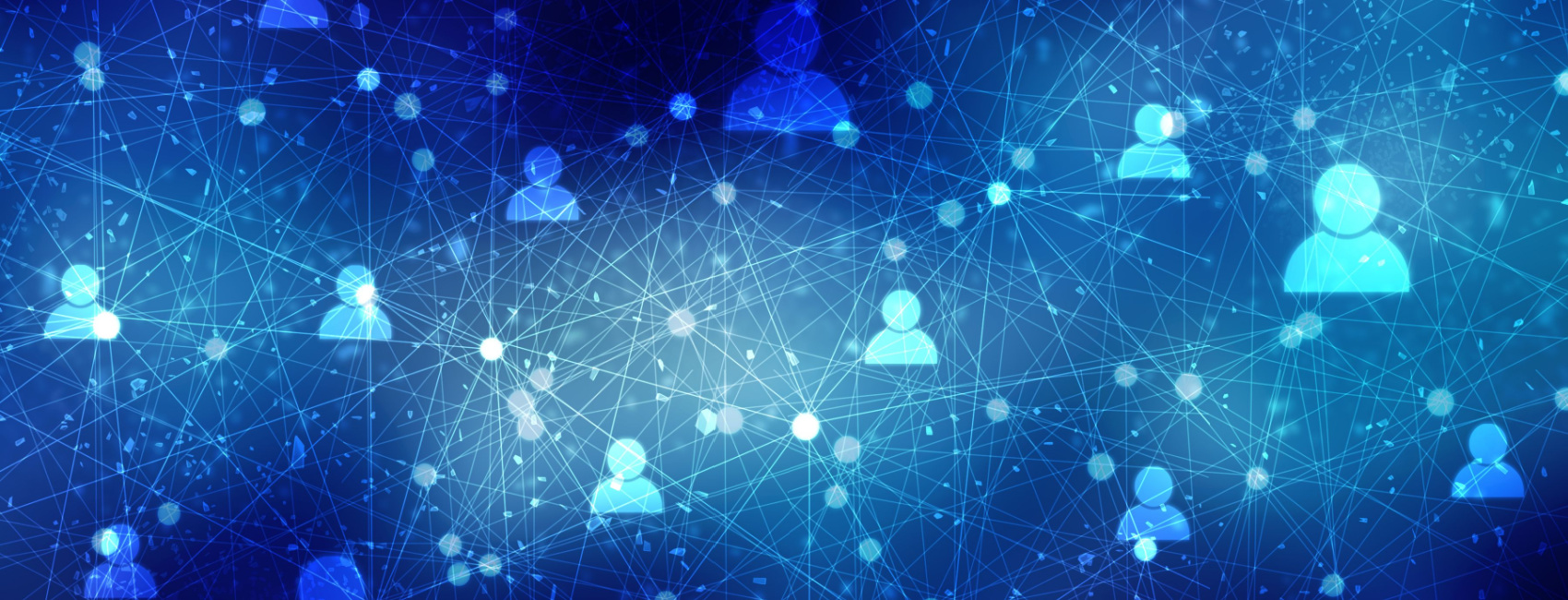
Applied Microeconomics
The Applied Microeconomics research group unites researchers working on a broad array of topics within such areas as labour economics, economics of education, health economics, family economics, urban economics, environmental economics, and the economics of science and innovation. The group operates in close collaboration with the CAGE Research Centre.
The group participates in the CAGE seminar on Applied Economics, which runs weekly on Tuesdays at 2:15pm. Students and faculty members of the group present their ongoing work in two brown bag seminars, held weekly on Tuesdays and Wednesdays at 1pm. Students, in collaboration with faculty members, also organise a bi-weekly reading group in applied econometrics on Thursdays at 1pm. The group organises numerous events throughout the year, including the Research Away Day and several thematic workshops.
Our activities
Work in Progress seminars
Tuesdays and Wednesdays 1-2pm
Students and faculty members of the group present their work in progress in two brown bag seminars. See below for a detailed scheduled of speakers.
Applied Econometrics reading group
Thursdays (bi-weekly) 1-2pm
Organised by students in collaboration with faculty members. See the Events calendar below for further details
People
Academics
Academics associated with the Applied Microeconomics Group are:
Research Students
Events
Econometrics Seminar - Michal Kolesar (Princeton)
Title: The Fragility of Sparsity" (with Ulrich Müller and Sebastian Roelsgaard) There is no paper yet, but the abstract is:
We argue, using three empirical applications, that linear regression estimators which rely on the assumption of sparsity are fragile in two ways. First, we document that different choices of the regressor matrix that don't impact long regression estimates, such as the choice of baseline category with categorical controls, move the post-double-selection estimates by one standard error or more. Second, we develop two tests of the sparsity assumption based on comparing sparsity-based estimators with long regression. Both tests tend to reject the sparsity assumption in all three applications. Unless the number of regressors is comparable to or exceeds the sample size, long regression yields more robust results at little efficiency cost.